
The Rise of Open Source LLMs
While powerful cloud-based Large Language Models (LLMs) raise significant privacy concerns due to potential third-party data storage and usage, open-source LLMs offer a privacy-centric alternative. By allowing users to run models locally or on their own private infrastructure, open-source options provide crucial benefits like complete data sovereignty (keeping data in-house), transparency through accessible code, offline capabilities, and customization potential.
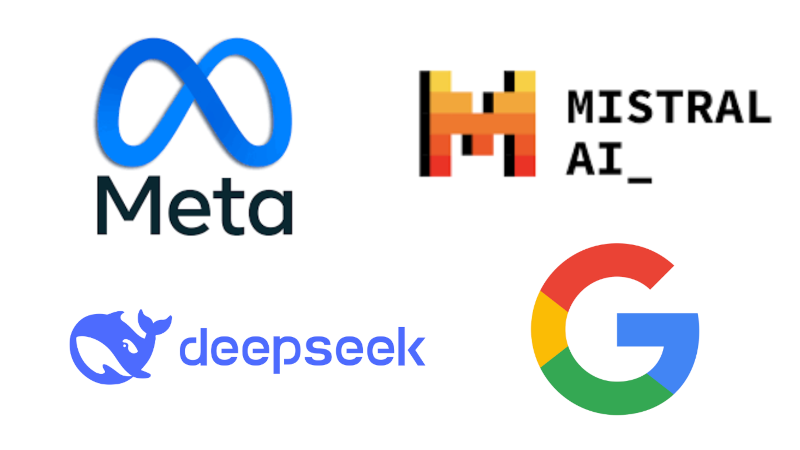
Artificial intelligence, particularly Large Language Models (LLMs) like ChatGPT, Claude, and Gemini, has captured the world's imagination. These powerful tools can write code, draft emails, summarize complex documents, and even create poetry. But as we integrate them more deeply into our personal and professional lives, a crucial question arises: What happens to our data?
When you use many popular, cloud-based AI services, your prompts, the data you input, and sometimes even the generated responses might be stored, analyzed, or used for further training by the provider. For individuals handling personal thoughts or businesses processing sensitive client information, this can be a significant privacy concern. Sending proprietary code, confidential strategic plans, or personal journals to a third-party server, even an AI one, involves a degree of trust and potential risk.
But what if there was another way? What if you could harness the power of LLMs without sending your data across the internet? Enter the exciting world of open-source LLMs.
What Are Open Source LLMs?
Unlike proprietary models developed and controlled by single companies, open-source LLMs have their underlying code, and often their architecture and even trained model weights, publicly available. This means anyone (with the right skills and resources) can inspect, modify, deploy, and run these models on their own infrastructure. Think of it like the difference between using a locked-down cloud software service versus installing and running open-source software like Linux or LibreOffice on your own computer.
Popular examples include models from families like Llama (Meta), Mistral (Mistral AI), Falcon (TII), and many others developed by research institutions and the vibrant open-source community.
The Privacy Advantage: Taking Back Control
This open nature directly translates into significant privacy benefits:
-
Data Sovereignty: This is the most crucial advantage. When you run an open-source LLM locally on your own computer or on private servers within your organization's network, your data never leaves your control. Your prompts and the information you process stay entirely within your trusted environment. There's no transmission to external servers, eliminating the risk of third-party data breaches or unintended data usage.
-
Transparency: Open-source models allow for scrutiny. Researchers, developers, and security experts can examine the code and architecture to understand how the model works and identify potential vulnerabilities. While understanding the exact reasoning behind every output of an LLM is still complex, the transparency is far greater than with proprietary "black box" models.
-
Offline Capability: Many open-source LLMs can be configured to run entirely offline once downloaded and set up. This is perfect for situations where internet connectivity is unreliable or where maximum security dictates an air-gapped environment. You can leverage AI capabilities without any external network communication.
-
Customization for Security: Need to fine-tune a model specifically on your company's internal (but non-sensitive) documentation without exposing that data structure externally? Or perhaps modify the model's behavior to strictly avoid generating certain types of content? Open source provides the flexibility to tailor the model to specific needs, potentially including enhanced security protocols or data handling rules.
Use Cases Where Privacy Matters
Imagine the possibilities:
- Developers: Analyzing or generating proprietary code snippets without uploading potentially sensitive intellectual property.
- Healthcare Professionals: Summarizing de-identified patient notes or querying medical research papers locally (always adhering to strict healthcare data regulations like HIPAA).
- Legal Teams: Reviewing confidential case documents or contracts within the firm's secure network.
- Researchers: Analyzing sensitive datasets without risking exposure.
- Individuals: Journaling, brainstorming personal ideas, or drafting sensitive emails without concerns about cloud storage.
Things to Consider
Of course, running open-source LLMs isn't without its challenges:
- Resource Requirements: Running larger, more capable models often requires significant computational power, particularly powerful GPUs and substantial RAM, which can be costly.
- Technical Expertise: Setting up, maintaining, and potentially fine-tuning these models requires technical knowledge. It's generally not a simple plug-and-play experience like cloud services.
- Performance: While open-source models are rapidly improving, the absolute cutting-edge performance might still reside with the largest proprietary models, though the gap is narrowing significantly.
The Future is Open (and Private)
The open-source AI movement is incredibly dynamic. Models are becoming more capable, efficient, and easier to run on consumer-grade hardware. The community is constantly innovating, providing tools and support that lower the barrier to entry.
For individuals and organizations prioritizing data privacy and control, open-source LLMs offer a compelling and increasingly viable alternative to proprietary cloud services. They empower users to leverage the transformative power of AI without compromising on data security. It's an exciting development that puts control back where it belongs: in your hands.
So, next time you think about using an LLM for a sensitive task, consider exploring the open-source landscape. You might find the perfect balance of power and privacy.